Hey ya’ll! Probability theory and random variables come up all the time in machine learning. Classification techniques like Naive Bayes, the likelihood ratio test and maximum a posterior (MAP). A lot of times when someone says “Naive Bayes classification” they imply that they want to assume that the data is multinomial (counts from a fixed dictionary) or when they say “MAP classification” they mean they assumed Gaussian distributions for each of the classes. In reality though the choice of these distributions is flexible and assuming different distributions in the PRT is easy thanks to the RV objects. This is the first post in a series that will highlight how RV objects can be used for rapid classifier generation and showcase some of the ways that we use RVs for our research. In part 1, we are going to give an overview of some of the basic RV objects and show how they are used in some basic classification techniques.
Contents
RV objects
Admittedly, the RV objects are one of the most under-documented features in the PRT. Sorry about that. I can take the blame there. Hopefully this post gets us started on fixing that.
Random variable objects are used to state that data is a random variable with an assumed distribution. Therefore, each prtRv*() assumes a different probability density function and implements the necessary methods to infer the parameters of the probability density function mle(), draw data with the same distribution draw(), and evaluate the likelihood of other data pdf() and logPdf().
Let’s make an RV object with a specified distribution. For the sake of this example we will use the multi-variate Normal distribution “MVN”.
rv = prtRvMvn('mu',[1 2],'sigma',[1 0.5; 0.5 1])
rv = prtRvMvn Properties: name: 'Multi-Variate Normal' nameAbbreviation: 'RVMVN' isSupervised: 0 isCrossValidateValid: 1 covarianceStructure: 'full' mu: [1 2] sigma: [2x2 double] plotOptions: [1x1 prtOptions.prtOptionsRvPlot] verboseStorage: 1 showProgressBar: 1 isTrained: 0 dataSetSummary: [] dataSet: [] userData: [1x1 struct]
Let’s draw some data from this RV object and put it into a prtDataSetClass().
x = rv.draw(1000); ds = prtDataSetClass(x);
Using this RV we can evaluate the log of the probability density function of the data that we drew.
y = rv.logPdf(x);
RV objects also have some plot methods and plotLogPdf() is probably the most useful. Let’s plot the log of the probability density function with the data that we drew fromt the pdf.
rv.plotLogPdf() hold on plot(ds); hold off
Although RV objects can be used by specifying the parameters of the densities their true power is flexibly modeling data. For example, let’s make another RV MVN object without specifying parameters and use it to estimate the parameters of the data we drew. Here we will estimate the parameters using maximum likelihood estimation mle()
rv2 = prtRvMvn;
rv2 = rv2.mle(ds); % or rv2 = rv2.mle(x);
estimatedMean = rv2.mu
estimateCovariance = rv2.sigma
estimatedMean = 0.9647 1.9896 estimateCovariance = 0.9479 0.4587 0.4587 0.9858
RV objects are actually sub-classes of prtActions() just like classifiers and regressors. This means that they have the train() and run() methods and can be cross-validated. By default, all RV objects implement train by calling the mle() method and implement run by using the logPdf() method. Therefore, some of the things we did above can be done as follows.
rv2 = rv2.train(ds); y = rv2.run(ds);
Types of RV objects
A list of available RVs that ship with the PRT can be displayed
dirContents = what(fullfile(prtRoot,‘rv’));
availableRvs = dirContents.m
availableRvs = ‘prtRv.m’ ‘prtRvDiscrete.m’ ‘prtRvGmm.m’ ‘prtRvHmm.m’ ‘prtRvIndependent.m’ ‘prtRvKde.m’ ‘prtRvMemebershipModel.m’ ‘prtRvMixture.m’ ‘prtRvMultinomial.m’ ‘prtRvMvn.m’ ‘prtRvUniform.m’ ‘prtRvUniformImproper.m’ ‘prtRvVq.m’
As you can, most of the standard probability densities have been implemented. In addition to standard things like prtRvMvn, prtRvDiscrete and prtRvMultinomial, there are also a few RVs that operate on other RVs like prtRvIndependent, prtRvMixture, prtRvGmm and prtRvHmm and there are a few RVs that can be used for more flexible density modeling like prtRvKde and prtRvVq. We will talk about some of these more advanced RVs in a later post.
Using RV Objects in Classifiers
There are two primary classifiers that make use of RV objects prtClassMap and prtClassGlrt. These classifiers have very similar performance but prtClassMap is able to handle M-ary classification problems so we will use that as our example.
class = prtClassMap
class = prtClassMap Properties: name: 'Maximum a Posteriori' nameAbbreviation: 'MAP' isNativeMary: 1 rvs: [1x1 prtRvMvn] twoClassParadigm: 'binary' internalDecider: [] isSupervised: 1 isCrossValidateValid: 1 verboseStorage: 1 showProgressBar: 1 isTrained: 0 dataSetSummary: [] dataSet: [] userData: [1x1 struct]
prtClassMap has a property rvs that lists the rvs used for each class in the incoming data set. If there is only one RV specified it is used to model all of the classes. Let’s classify prtDataGenUnimodal using a quadratic classifier that arises by using a MAP classifier with MVN assumption for each class.
class = prtClassMap('rvs',prtRvMvn);
ds = prtDataGenUnimodal;
trainedClassifier = class.train(ds);
plot(trainedClassifier);
If our data is more complex, we can modify the assumptions of the distributions in both of our classes by setting the “rvs” parameter to something more flexible. Let’s classify prtDataGenBimodal using prtRvKde which uses kernel density estimation.
class = prtClassMap(‘rvs’,prtRvKde); ds = prtDataGenBimodal;trainedClassifier = class.train(ds); plot(trainedClassifier);
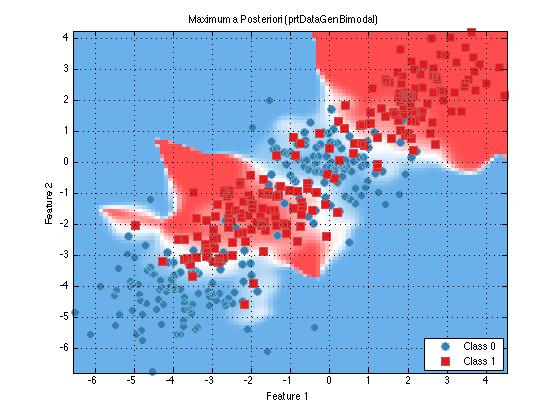
Conclusions
As you can see, RVs are pretty powerful parts of the PRT and they can be used in other parts of the PRT to make things flexible.
In future posts we will talk about how RV objects are used to make flexible mixtures like the GMM and hidden Markov models and we will explore some things that are still in beta such as how we use prtBrv objects to perform variational Bayesian inference for models like Dirichlet process mixtures.